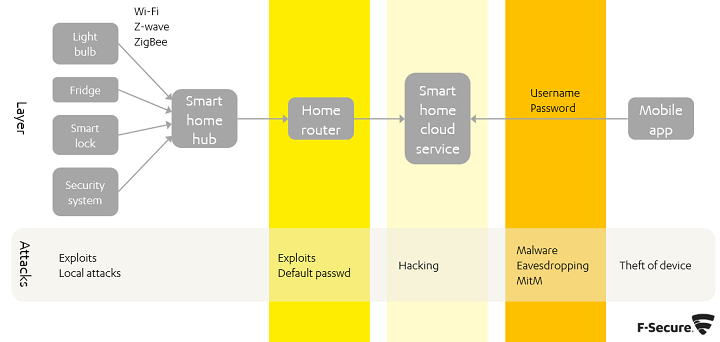
Image: The above image shows a typical smart home configuration and the kinds of attacks it can face. While the smart home is not a target at the moment due to its low adoption rate and high fragmentation, all of the layers can be attacked with existing techniques.
If you are extremely worried about your privacy and security, the only way to really stay safe is to not buy and use these gadgets. However, for most people, the time-saving convenience benefits of IoT and the Smart Home will outweigh most privacy and security implications. Also, IoT devices are not widely targeted at the moment and even when they are, the attackers are after the computing power of the device -- not yet your data or your home. Actually, the biggest risk right now comes from the way how the manufacturers of these devices handle your personal data. This all said, you shouldn't just blindly jump in. There are some things that you can do to reduce the risks:
• Do not connect these devices directly to public internet addresses. Use a firewall or at least a NAT (Network Address Translation) router in front of the devices to make sure they are not discoverable from the Internet. You should disable UPnP (Universal Plug and Play) on your router if you want to make sure the devices cannot open a port on your public internet address.
• Go through the privacy and security settings of the device or service and remove everything you don't need. For many of these devices the currently available settings are precious few, however. Shut down features you don't need if you think they might have any privacy implications. For example, do you really use the voice commands feature in your Smart TV or gaming console? If you never use it, just disable it. You can always enable it back if you want to give the feature a try later.
• When you register to the cloud service of the IoT device, use a strong and unique password and keep it safe. Change the password if you think there is a risk someone managed to spy it. Also, as all of these services allow for a password reset through your email account, make sure you secure the email account with a truly strong password and keep the password safe. Use 2-factor authentication (2FA) where available -- and for most popular email services it is available today.
• Keep your PCs, tablets, and mobile phones clear of malware. Malware often steals passwords and may hence steal the password to your smart home service or the email account linked to it. You need to install security software onto devices where you use the passwords, keep your software updated with the latest security fixes, and, as an example, make sure you don't click on links or attachments in weird spam emails.
• Think carefully if you really want to use remotely accessible smart locks on your home doors. If you're one of those people who leave the key under the door mat or the flower pot, you're probably safer with a smart lock, though.
• If you install security cameras and nannycams, disconnect them from the network when you have no need for them. Consider doing the same for devices that constantly send audio from your home to the cloud unless you really do use them all the time. Remember that most IoT devices don't have much computing power and hence the audio and video processing is most likely done on some server in the cloud.
• Use encryption (preferably WPA2) in your home Wi-Fi. Use a strong Wi-Fi passphrase and keep it safe. Without a passphrase, with a weak passphrase, or when using an obsolete protocol such as WEP, your home Wi-Fi becomes an open network from a security perspective.
• Be careful when using Open Wi-Fi networks such as the network in a coffee shop, a shopping mall, or a hotel. If you or your applications send your passwords in clear text, they can be stolen and you may become a victim of a Man-in-the-Middle (MitM) attack. Use a VPN application always when using Open Wi-Fi. Again, your passwords are they key to your identity and also to your personal Internet of Things.
• Limit your attack surface. Don't install devices you know you're not going to need. Shut down and remove all devices that you no longer need or use. When you buy a top of the line washing machine, and you notice it can be connected through Wi-Fi, consider if you really want and need to connect it before you do. Disconnect the device from the network once you realize you actually don't use the online features at all.
• When selecting which manufacturer you buy your device from, check what they say about security and privacy and what their privacy principles are. Was the product rushed to the market and were any security corners cut? What is the motivation of the manufacturer to process your data? Do they sell it onwards to advertisers? Do they store any of your data and where do they store it?
• Go to your home router settings today. Make sure you disable services that are exposed to the Internet -- the WAN interface. Change the admin password to something strong and unique. Check that the DNS setting of the router points to your ISP's DNS server or some open service like OpenDNS or Google DNS and hasn't been tampered with.
• Make sure you keep your router's firmware up-to-date and consider replacing the router with a new one, especially, if the manufacturer no longer provides security updates. Consider moving away from a manufacturer that doesn't do security updates or stops them after two years. The security of your home network starts from the router and the router is exposed to the Internet.
The above list of actions is extensive and maybe a bit on the "band-aid on the webcam"-paranoid side. However, it should give you an idea of what kinds of things you can do to stay in control of your security and privacy when taking a leap to the Internet of Things. Security in the IoT World is not that different from earlier: Your passwords are also very important in IoT as is the principle of deploying security patches and turning off services you don't need.